Bureau Waardenburg (BUWA; https://www.buwa.nl) is a SPECTORS partner who specializes in the fields of ecology, environment and landscape design, commissioned by both governments and private companies. Inside the SPECTORS project, BUWA focuses on developing products for monitoring nature and water.
Identifying Caltha palustris from aerial images using deep learning
Unmanned aerial vehicles (UAVs) have been increasingly used in the area of vegetation mapping because they are ideal for acquiring data in areas like swamps or steep slopes that are hard to access. On top of this, professional deployment of UAVs with high-resolution sensors and the developments in computer vision provides the possibility to capture and analyse detailed images in ways that were previously impossible. As part of the SPECTORS project, BUWA explores the potential of deep learning for plant species recognition from UAV images, in order to assist or replace labour and cost-intensive field inventories.
Inspired by the work of Hochschule Rhein-Waal (HSRW) and in collaboration with the Wageningen University & Research (WUR), a convolutional neural network (CNN) based algorithm had been developed to identify plant species using the images collected by a DJI Phantom 4 Pro+. The target species is Caltha palustris (also known as “Marsh Marigolds”), which is a native plant species in Dutch wetlands with distinct bright yellow flowers that blossom in the early spring.
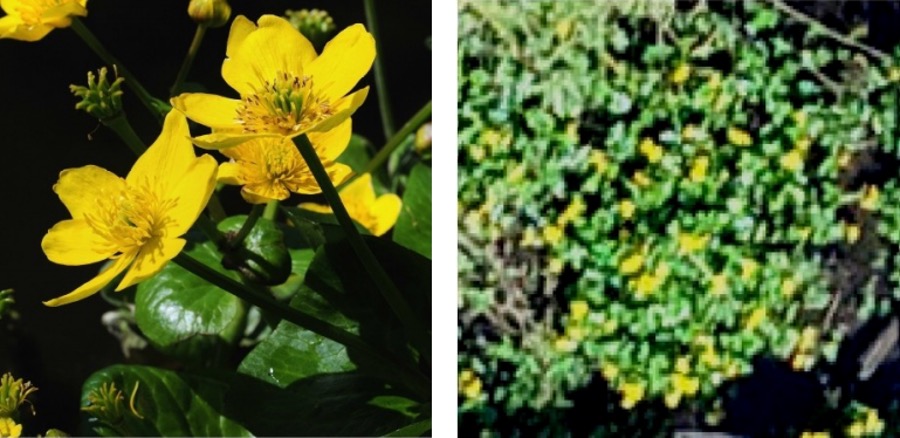
A field campaign was conducted in the Biesbosch National Park, the Netherlands. Training data was generated from the UAV flights over open grasslands and willow forests. Two CNN models were used for comparison – one produced a single classification of Caltha palustris per sample, while the other model predicted the presence of Caltha palustris on a 16×16 grid overlaid each sample for better localization. Initial results demonstrated that the single predictions outperformed the grid predictions, and that the model was capable to predict the presence of Caltha palustris in the forest area reliably. In the future, a dedicated machine will be developed to run the process of classification much faster or an existing model would be fine-tuned to improve the accuracy and speed. We are also developing other applications for the deep learning technique (non aerial photo recognition).
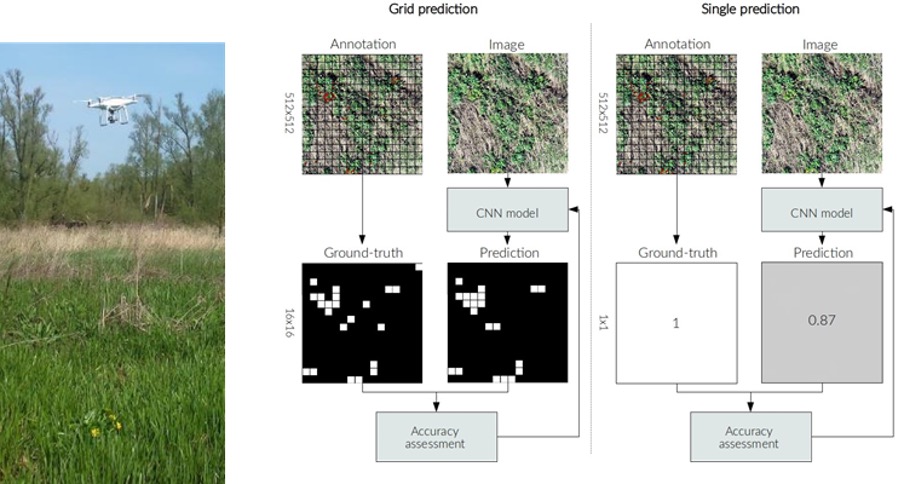
Monitoring vegetation and structure with remote sensing
Again together with WUR, an operational service that uses remote sensing technologies to support vegetation mapping was being set up. The developed system makes use of the national satellite imagery, aerial photographs and LiDAR data to monitor different dominant vegetation species and to determine the vegetation heights, while using drone data for validation purposes. This service will support the Province of Gelderland in nature management and monitoring of Natura 2000 and the Dutch Integrated Approach to Nitrogen.
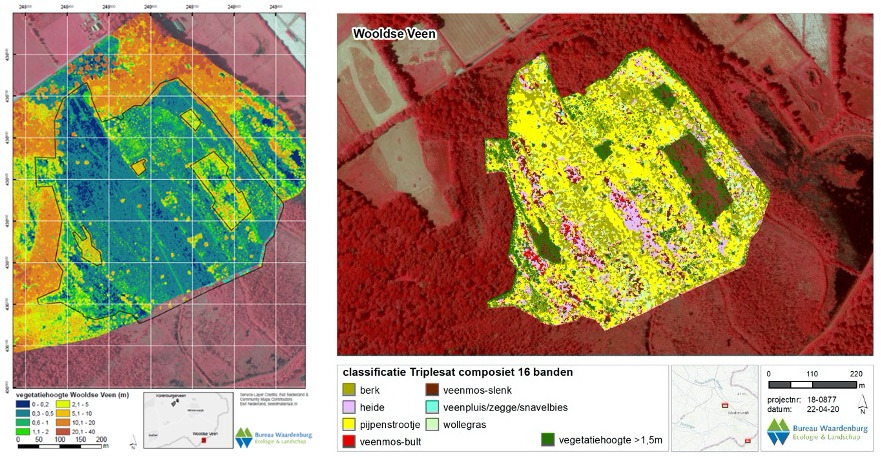