KWR Water Research Institute (http://www.kwrwater.nl) is one of the SPECTORS partners that focuses on creating high quality vegetation maps using images of Remotely Piloted Aircraft Systems (RPAS, commonly known as “drones”).
Object recognition and vegetation mapping
KWR developed an object recognition workflow to convert Remote sensing images (by RPAS or satellites) into a number of pre-defined vegetation classes. An accurate vegetation map is not only important for nature management, but also to quantify evaporation and thus groundwater recharge as well as possbile.
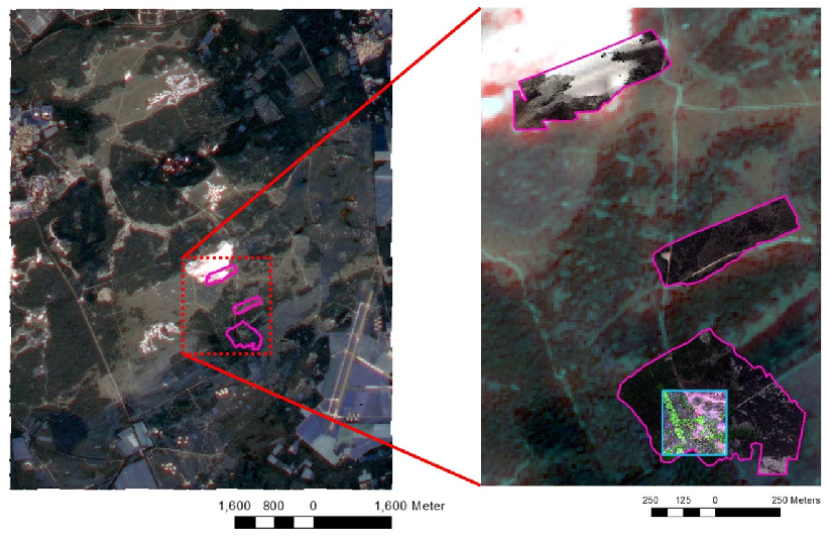
KWR used the “patch-based feature extraction” method to classify the acquired images, and developed algorithms to automate texture analysis and feeding the results into a “Random Forest” classification method. The aim was to distinguish the vegetation classes: “bare ground”, “grassland”, “shrubs”, “coniferous forest”, “deciduous forest”, and “mixed forest”.
Challenges
Object recognition is no easy task, various steps and components were necessary to create a well-founded workflow. Starting with correctly setting up the sensors and the flight missions to obtain sharp and undistorted images for creating an ortho-mosaic image. Then preparing the baseline samples, including the ground-truthing data for training, validation and testing. Followed by the conversion of raw pixel-based images into digital maps that are automatically divided into pre-defined vegetation classes. And finally, verifying and evaluating the results using reference data to determine the quality of the output.
Current status
KWR has developed an entire workflow of image acquisition, data processing and object recognition. On top of this, the classification methods are proven to be practically useful for automated vegetation mapping using the acquired RPAS images. Figure below shows the result of this application, which is a reliable representation of different vegetation classes and can be used for monitoring and management of the (nature) area.
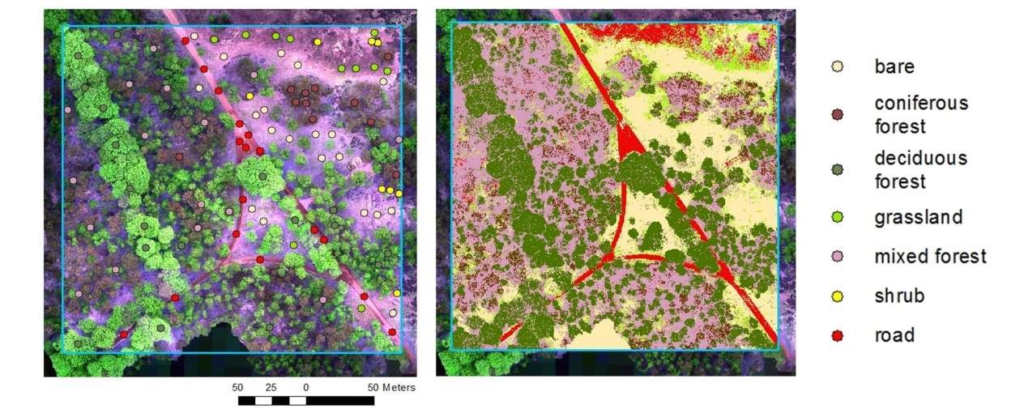
Future steps
Now that the conversion of RPAS images into vegetation classes has been successful, KWR plans to further investigate other potential applications using a similar approach, for example determining the number of trees, coverage of vegetation classes or maybe even of (larger) individual plant species.
References
KWR. (n.d.) INTERREG ‘SPECTORS’. [Online] Available at: https://www.kwrwater.nl/en/projecten/interreg-spectors/, Accessed on: 10th July, 2019
Dorland, E., Vonk, E., Fujita, Y., Raterman, B. & Witte, F. (2018) Van dronebeelden naar vegetatieklassen: een methode voor objectherkenning. In: H2O-Online, 1-6